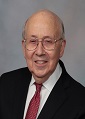
Richard Weinshilboum
Richard Weinshilboum Professor of Pharmacology and Medicine Mayo Clinic Rochester, MN, USA
Title: Pharmaco-omics of Selective Serotonin Reuptake Inhibitor (SSRI) Response
Biography
Biography: Richard Weinshilboum
Abstract
Pharmacogenetics involves the study of the role of inheritance in individual variation in drug response phenotypes. In the 21st century “pharmacogenetics” has evolved to become “pharmacogenomics” with our ability to scan agnostically across the genome and then to become “pharmaco-omics” with the merger of other “omics” techniques with genomics. We have applied a metabolomics-informed genomics research strategy to study the pharmaco-omics of SSRIs, the class of drugs that is the worldwide standard of care for the pharmacotherapy of the number one psychiatric disease, Major Depressive Disorder (MDD, depression). Specifically, we identified metabolites assayed with an LCECA platform that were associated with measures of outcomes (HAMD and QUIDS) in response to the SSRI therapy of MDD patients enrolled in the 803 patient Mayo Clinic PGRN-AMPS SSRI clinical trial. The assayed metabolite that was most highly associated with outcomes (remission and response) was plasma serotonin (5-HT) and the metabolite most highly associated with symptom severity was plasma kynurenine. GWAS for plasma 5-HT identified two genome-wide significant SNP signals near or across the TSPAN5 and ERICH3 genes, while GWAS for plasma kynurenine identified SNPs in the DEFB1 and AHR genes. Subsequent functional genomic studies showed that knockdown of TSPAN5 and ERICH3 in CNS-derived cells resulted in decreased 5-HT in the culture media, that ERICH3 played a role in monoamine vesicular function and that DEFB1 and AHR were both associated with inflammation, a process that contributes to MDD pathophysiology. Finally, a machine-learning based predictive algorithm was developed that incorporated these SNPs which could predict SSRI response with approximately 75% accuracy as compared with 55% using only clinical information. In summary, the application of a “pharmaco-omic” research strategy identified novel genes that contribute to individual variation in SSRI therapeutic response and also, perhaps, to mechanisms involved in MDD pathophysiology.